머신 러닝과 차원 축소 dimension reduction
- 피처들이 늘어날수록 데이터간의 거리는 크게 증가
- 중요하지 않은 피처가 많은 데이터의 경우 그렇지 않은 경우보다 예측 정확도가 떨어짐
+ 피처 간의 상관 관계의 영향(다중 공선성)
=> 차원 축소 실시
차원 축소
- 특징 추출 feature exraction : 기존의 데이터셋을 개선된 형태로 축소된 공간으로 맵핑
- 특징 선택 feature selection : 기존의 데이터셋에서 중요한 특징들만 선정
=> PCA, SVD, NMF 등
- 이미지나 텍스트에서 차원 축소이 성능 향상에 도움이 됨.
주성분 분석 Principal Component Analysis
- 특징들의 공분산 행렬로부터 주성분을 추출하는 방법
- 공분산 행렬에서 직교화된 고유값과 고유 행렬을 구함. 고유값은 해당 번째 주성분의 중요도, 고유 벡터는 해당번째 주성분의 가중 계수벡터
- 서로 다른 고유 벡터는 직교하므로 두 주성분 사이 공분산은 0, 고유 벡터는 자기 자신의 전치한것과 내적은 1
붓꽃 데이터로 살펴보자
일단 데이터 준비
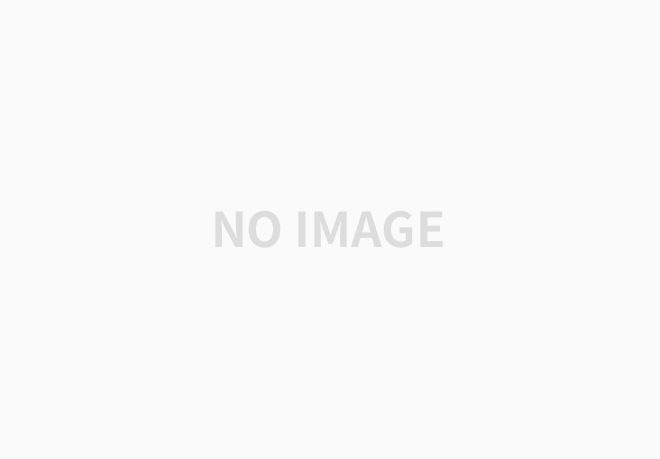
from sklearn.datasets import load_iris
import pandas as pd
import matplotlib.pyplot as plt
iris = load_iris()
cols = ["sepal_length", "sepal_width", "petal_length", "petal_width"]
df = pd.DataFrame(data=iris.data, columns=cols)
df["target"]=iris.target
df.head()
데이터를 간단하게 살펴보면
sepal_length와 sepal_width 만으로도 어느정도 붓꽃의 종류를 분류할수 있음을 알수있다.
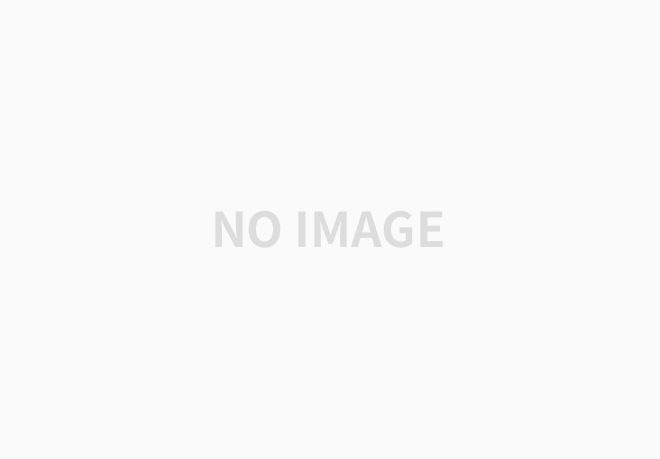
markers = ["^", "s", "o"]
for i, marker in enumerate(markers):
x_val = df[df["target"] ==i]["sepal_length"]
y_val = df[df["target"]==i]["sepal_width"]
plt.scatter(x_val, y_val, marker=marker,label=iris.target_names[i])
plt.legend()
plt.xlabel("sepal_length")
plt.ylabel("sepal_width")
plt.show()
주성분 분석은 여러 피처들을 같이 다루므로
사전에 표준화부터 시켜주어야 한다.
표준화 후 기존의 피처 4개를 2개의 주성분으로 축소시키자.
from sklearn.preprocessing import StandardScaler
from sklearn.decomposition import PCA
df_scaled = StandardScaler().fit_transform(df)
pca = PCA(n_components=2)
pca.fit(df_scaled)
df_pca = pca.transform(df_scaled)
print(df_pca.shape)
표준화나 pca 변환 후에는 넘파이 배열 형태로 되어있으므로
주성분 변환 결과를 데이터 프레임으로 변환시킨 후
주성분 1을 x축 주성분 2를 y축으로 할때 어떻게 분류되는지 시각화하여보자
두개의 주성분 만으로 대부분의 데이터를 올바르게 분류하고 있는걸 확인할 수 있다.
마지막에 pca.explained_variance_ratio_로
해당 주성분들이 데이터를 얼마나 잘 설명하고 있는지 나타내고 있으며
주성분요소 1은 76%, 주성분 요소 2는 18%정도로 전체 변동에서의 비율을 나타내고 있다.
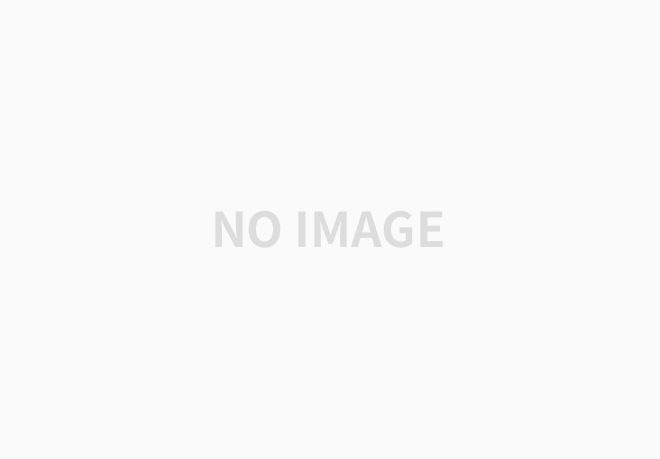
cols = ["pc1", "pc2"]
df_pca = pd.DataFrame(data=df_pca, columns=cols)
df_pca["target"] = iris.target
markers = ["^", "s", "o"]
for i, marker in enumerate(markers):
x_val = df_pca[df_pca["target"] ==i]["pc1"]
y_val = df_pca[df_pca["target"]==i]["pc2"]
plt.scatter(x_val, y_val, marker=marker,label=iris.target_names[i])
plt.legend()
plt.xlabel("pc1")
plt.ylabel("pc2")
plt.show()
print(pca.explained_variance_ratio_)
랜덤 포레스트로
기존 데이터와 pca 적용 후 데이터 정확도를 살펴보았는데
pca 후가 다소 좋은 정확도를 얻음.
일반적으로 차원이 축소된 후(정보가 줄어들어) 예측 성능을 떨어짐
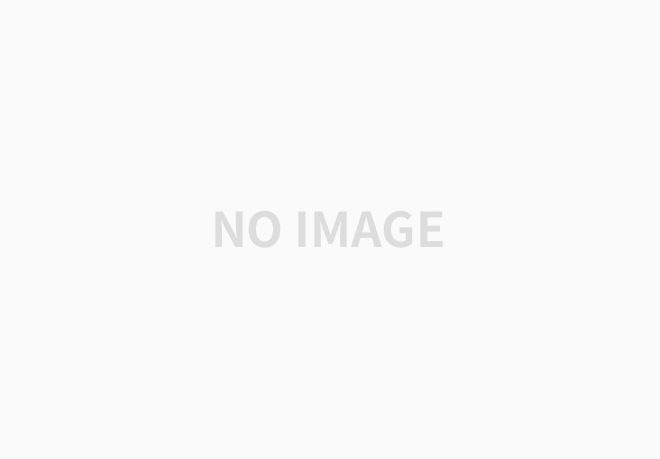
from sklearn.ensemble import RandomForestClassifier
from sklearn.model_selection import cross_val_score
import numpy as np
rf = RandomForestClassifier()
scores = cross_val_score(rf, iris.data, iris.target, scoring="accuracy",cv=3)
print("normal accuracy : {0:.3f}".format(np.mean(scores)))
scores = cross_val_score(rf, df_pca.iloc[:,:-1], df_pca.iloc[:,-1], scoring="accuracy", cv=3)
print("pca accuracy : {0:.3f}".format(np.mean(scores)))
'인공지능' 카테고리의 다른 글
파이썬머신러닝 - 29. 선형 판별 분석 (0) | 2020.12.10 |
---|---|
파이썬머신러닝 - 29. 신용 카드 데이터 분석하기 + PCA (0) | 2020.12.10 |
파이썬머신러닝 - 27. 고급 회귀 기법을 이용한 주택 가격 예측 (0) | 2020.12.10 |
파이썬머신러닝 - 26. 자전거 대여 수요 예측하기 (0) | 2020.12.08 |
파이썬머신러닝 - 25. 트리기반 회귀분석 (0) | 2020.12.08 |